UNIST(총장 박종래) 인공지능대학원 유재준 교수팀이 4일 개최된 세계적인 컴퓨터 비전 학회 ECCV(European Conference on Computer Vision) 2024에서 3편의 논문을 발표하며, AI 경량화에서 디자인 자동화까지 AI 기술의 미래를 제시했다.
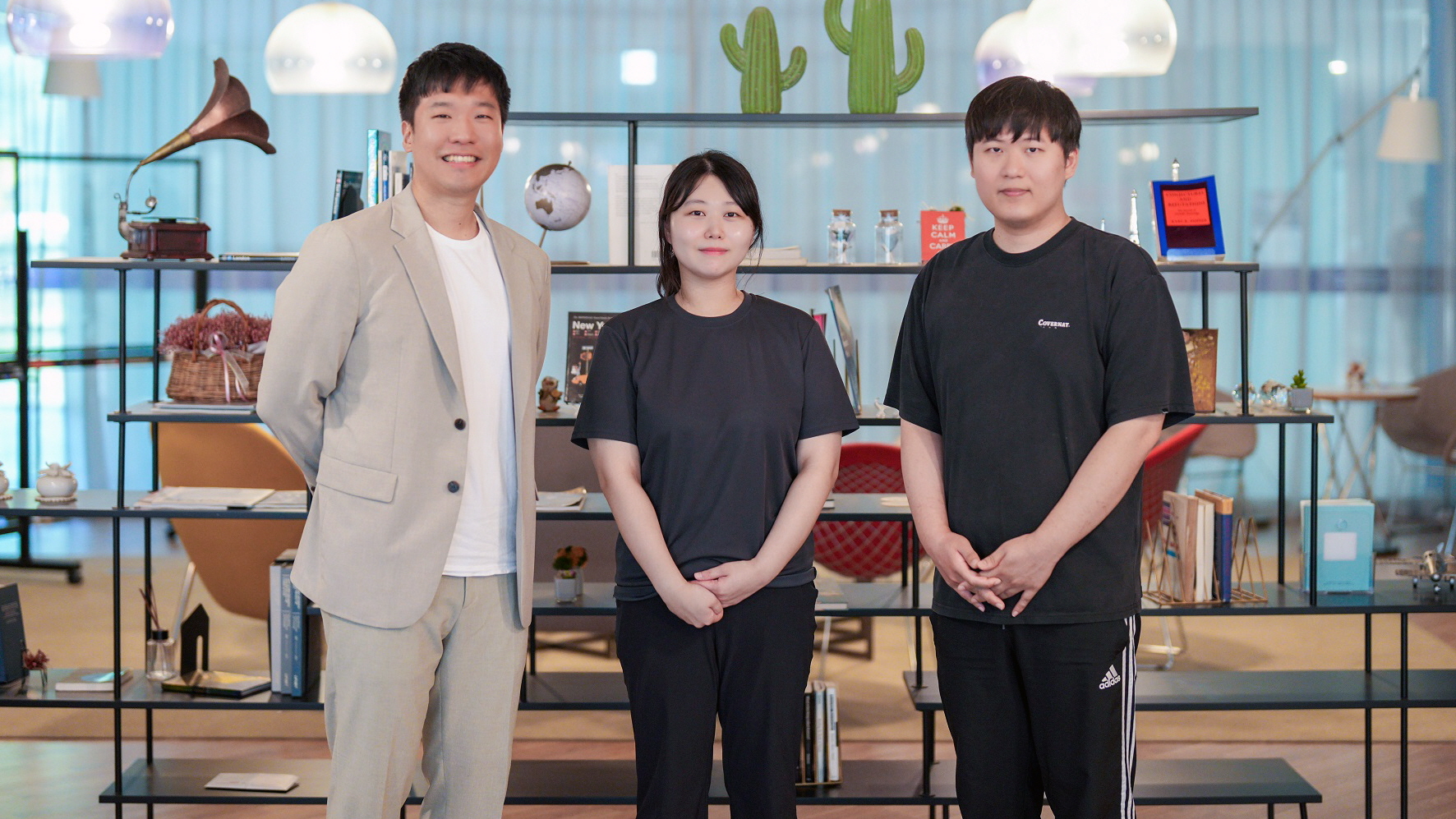
▲(왼쪽부터)유재준 교수, 장유진 연구원, 여상엽 연구원 등 AI 경량화 알고리즘 연구팀
UNIST 유재준 교수팀, 경량화·비디오·디자인 혁신 발표
생성형 AI를 323배 압축해도 고성능을 유지할 수 있는 기술이 개발돼 엣지 디바이스나 저전력 컴퓨터에서도 AI를 효율적으로 사용할 수 있는 가능성이 제시됐다.
UNIST(총장 박종래)는 인공지능대학원 유재준 교수팀이 4일 개최된 세계적인 컴퓨터 비전 학회 ECCV(European Conference on Computer Vision) 2024에서 3편의 논문을 발표하며, AI 경량화에서 디자인 자동화까지 AI 기술의 미래를 제시했다고 밝혔다.
유재준 교수팀은 이미지 생성 AI인 GAN(Generative Adversarial Networks)을 323배까지 압축하면서도 성능 저하 없이 경량화하는 데 성공했다.
지식 증류(knowledge distillation) 기법을 활용해 고성능 컴퓨터가 없는 엣지 디바이스나 저전력 컴퓨터에서도 AI를 효율적으로 사용할 수 있는 가능성을 제시했다.
연구팀은 DiME와 NICKEL 기법을 도입해 이미지 하나하나 비교하지 않고 분포를 비교하는 방식을 사용해 안정성을 높였다. 예를 들어, 선생 모델이 김태희 이미지를 만들면, 학생 모델이 송혜교나 전지현 이미지를 만들어도 학습이 가능하도록 한 것이다.
NICKEL 기법은 생성기와 분류기 간의 상호작용을 최적화해, 경량화된 모델에서도 높은 성능을 유지하게 돕는다. 두 기법을 결합한 결과, 323배 압축된 GAN 모델도 기존과 동일한 수준의 고품질 이미지를 생성할 수 있었다.
유 교수는 “323배 압축된 GAN이 기존 수준의 고품질 이미지를 만들어낸다는 점을 입증했다”며 “엣지 컴퓨팅이나 저전력 디바이스에서도 고성능 AI를 사용할 수 있는 길을 열었다”고 말했다.
제1저자 여상엽 연구원은 “제한된 자원에서도 고성능 AI 구현 가능성을 열어 AI 활용 범위를 크게 확장할 것”이라고 설명했다.
또한 유재준 교수팀은 고성능 컴퓨팅 자원이 부족한 환경에서도 고해상도 영상을 효율적으로 생성할 수 있는 하이브리드 비디오 생성 모델(HVDM)을 개발했다. HVDM은 2D 트리플레인 표현과 3D 웨이블릿 변환을 결합해, 영상의 전역적 맥락과 세밀한 디테일을 동시에 처리할 수 있다.
기존 비디오 생성 모델들은 고성능 컴퓨팅 자원에 의존해 고해상도 영상을 생성했지만, HVDM은 제한된 자원으로도 자연스럽고 고품질의 영상을 구현하는 데 성공했다. CNN 기반 오토인코더 방식이 가지는 한계를 극복한 것이다.
연구팀은 UCF-101, SkyTimelapse, TaiChi 등의 비디오 벤치마크 데이터셋을 통해 HVDM의 우수성을 입증했다. HVDM은 기존 기술보다 더 높은 비디오 품질을 구현하며, 자연스러운 영상 흐름과 사실적인 디테일에서 뛰어난 성능을 보여줬다.
유 교수는 “HVDM은 고성능 컴퓨팅 자원이 부족한 상황에서도 고해상도 비디오를 효율적으로 생성할 수 있는 획기적인 모델”이라며 “비디오 제작 및 시뮬레이션 같은 산업 분야에서 널리 활용될 수 있을 것”이라고 전했다.
여기에 연구팀은 적은 데이터로도 광고 배너와 Web-UI 디자인을 자동 생성할 수 있는 멀티모달 레이아웃 생성 모델도 개발했다.
이 모델은 이미지와 텍스트를 동시에 처리해 사용자 입력만으로 적절한 레이아웃을 자동 생성할 수 있다.
기존 모델들은 데이터 부족으로 텍스트와 이미지 정보를 충분히 처리하지 못했다. 새롭게 개발된 모델은 이 문제를 해결하며 광고 디자인과 웹 UI의 실용성을 크게 개선했다. 텍스트와 이미지 간 상호작용을 극대화해 시각적 요소와 텍스트를 동시에 반영한 최적화된 디자인을 자동으로 생성한다.
연구팀은 HTML 코드 형식으로 레이아웃 정보를 변환했다. 언어 모델의 사전 학습 데이터를 최대한 활용해 적은 데이터로도 뛰어난 성능을 발휘할 수 있는 자동 생성 파이프라인을 구축했다. 벤치마크 테스트 결과, 최대 2,800%의 성능 향상을 기록했다.
사전 학습 과정에서 이미지 캡션 데이터셋을 활용하고, Depth-Map과 ControlNet 기법을 결합해 데이터 증강을 통해 성능을 극대화했다. 레이아웃 생성의 품질이 대폭 개선됐으며, 데이터 전처리 과정에서 발생할 수 있는 왜곡을 줄여 자연스러운 디자인을 만들었다.
유 교수는 “5,000장 정도의 적은 데이터로도 기존 6만 장 이상의 데이터를 필요로 했던 모델보다 더 나은 성능을 발휘했다”며 “전문가뿐만 아니라 일반 사용자도 쉽게 사용할 수 있어 광고 배너와 웹 UI 디자인 자동화에 큰 혁신을 가져올 것”이라고 강조했다.
연구는 한국연구재단(NRF), 과학기술정보통신부(MSIT), 정보통신기획평가원(IITP), UNIST의 지원을 받아 수행됐다. 연구 성과는 다양한 산업 분야에서 AI 활용 가능성을 더욱 넓히며, 성능과 효율성을 극대화할 것으로 기대된다.