80개 넘는 차원에서 데이터 검사, 자체 학습 기능 제공
Appier는 자사의 네트워크에서 실시한 실제 연구 분석 결과를 공유하며 인공 지능 기반 모델의 부정 인스톨(Ad fraud) 방지 능력을 입증했다.
자사는 지난 2017년 5월부터 8월까지, 4개월 간 광고 클릭 및 앱 설치 정보 등 40억개 이상의 캠페인 데이터 포인트를 활용하여 연구를 진행했다. 이 연구를 통해 인공 지능을 기반으로 하는 모형이 기존 규칙 기반 모형에 비해 두 배 가량 빠른 속도로 부정 인스톨 패턴을 분석하는 것으로 나타났다.
인공 지능 기반 모형의 장점은 기존 모형이 감지하기 어려운 부정 인스톨 패턴을 찾아 낼 수 있다는 것이다. 예를 들어, Appier가 발견한 하나의 사기 패턴은 “카멜레온”인데, 이는 처음에 합법적인 광고물 퍼블리셔로 위장한 뒤 추후 부정 인스톨을 발생시키는 게시자를 의미한다. 또 다른 사기 패턴은 “재고 버스트”이다. 이 패턴을 통해 인스톨 광고주의 애플리케이션 상에서 적절한 수준의 인앱 활동이 이루어지지 않을 경우, 비정상적으로 높은 재고량을 집계하게 된다.
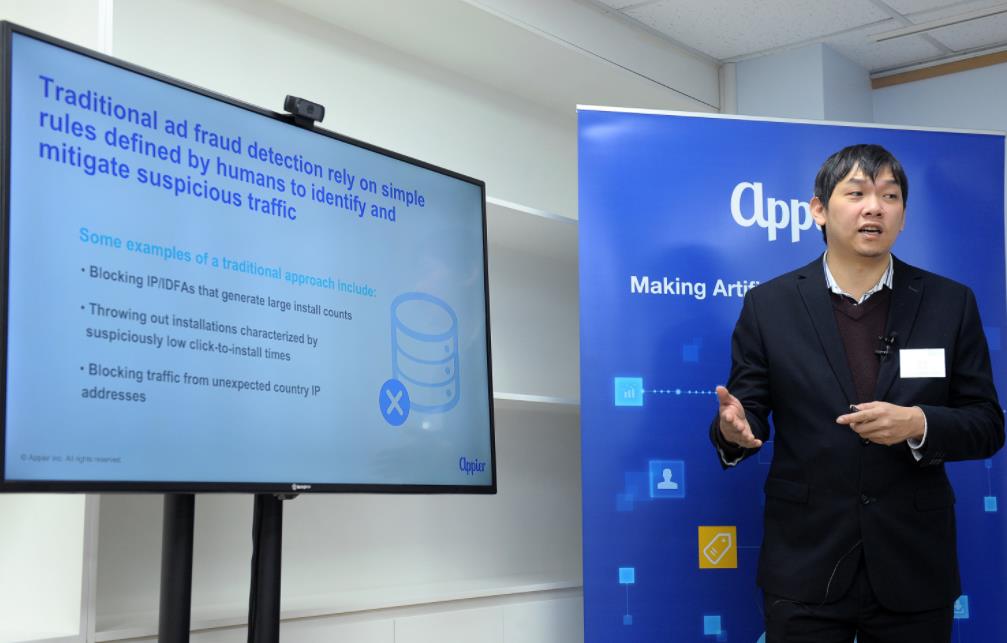
Appier의 최고 기술 책임자(CTO) 조 수(Joe Su)는 "부정 인스톨을 온라인 광고 업계에 큰 위협이 되었으며, 광고주들은 향후 수십억 달러의 비용을 발생할 것으로 예상됩니다"고 말했으며, "부정 인스톨을 찾아내고 그 영향을 최소화하는 기존의 규칙 기반 방법에는 한계가 있습니다. Appier는 인공 지능 기반 모형이 훨씬 더 효과적이라 믿으며, 실제로 네트워크를 분석 한 지 불과 4개월 만에 AI 접근 방식의 이점이 확인되고 있음을 알 수 있습니다.”라고 덧붙였다.
기존의 규칙 기반 모형은 통상 1~3개정도 차원만 보고, 인간이 프로그래밍한 규칙에 따라 이미 알려진 부정 인스톨 패턴으로 작동한다. 반면, 인공 지능 기반 모형은 80개가 넘는 차원에서 데이터를 검사 할 뿐만 아니라, 자체 학습 기능을 제공하기 때문에 과거에는 볼 수 없었던 새로운 의심 패턴을 감지할 수 있다.
또한 CTO조수는 "사이버 사기 또는 금융 사기와 마찬가지로 부정 인스톨이 더욱 정교해지고 끊임없이 진화하고 있기 때문에, 새로운 위협을 신속하게 식별하고 그 영향을 최소화 하는 것이 중요합니다. 기존의 규칙 기반 접근법을 사용하면 사기법들의 수법을 따라갈 수 없으며, 진화하는 부정 인스톨 패턴을 효과적으로 추적하기 위해서는 인공 지능 기반 모델이 필요합니다"고 덧붙였다.