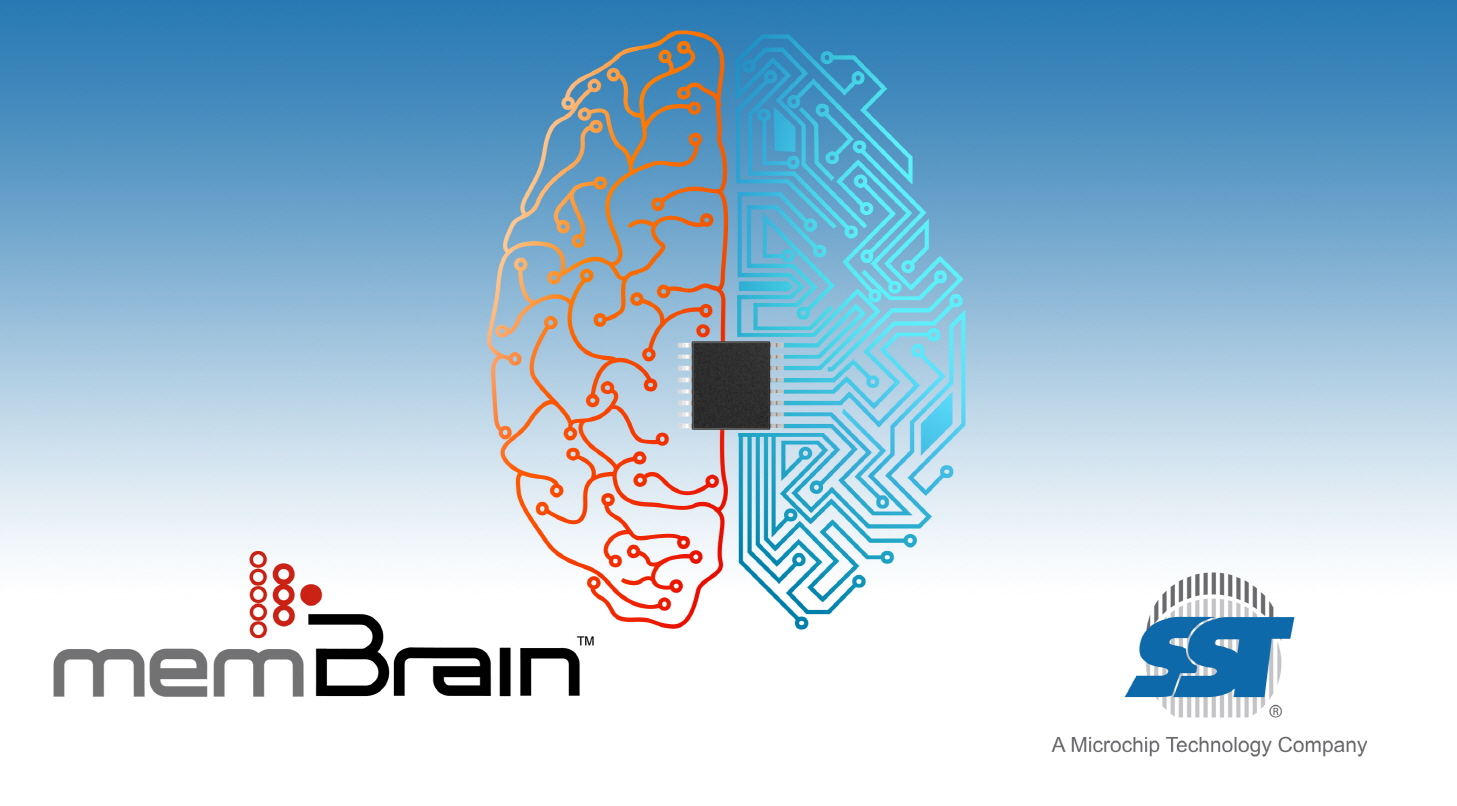
▲마이크로칩 자회사 SST와 인텔리전트 하드웨어 코리아 협업(이미지:마이크로칩)
마이크로칩의 memBrain NVM인메모리 컴퓨팅 기술 기반
IHWK사와 협업, 신경망기술 디바이스용 SoC 개발 진행
엣지 AI 가속화를 지원하는 컴퓨팅 플랫폼 개발을 위해 마이크로칩은 자회사 SST와 인텔리전트 하드웨어 코리아의 협업으로 시너지를 내고 있다.
마이크로칩테크놀로지(이하 마이크로칩)는 자회사인 실리콘 스토리지 테크놀로지(Silicon Storage Technology, SST)를 통해 SuperFlash memBrain 뉴로모픽 메모리 솔루션 평가에 사용되고 있는 시스템을 제공해 이 플랫폼 개발을 지원하고 있다고 14일 밝혔다.
이 솔루션은 업계에서 이미 검증받은 마이크로칩의 슈퍼플래시(SuperFlash) 기술 기반의 비휘발성 메모리(NVM: nonvolatile memory)를 기반으로 하고 있으며, 아날로그 방식의 인메모리 컴퓨팅 접근을 통해 뉴럴(neural) 네트워크를 위한 벡터 및 행렬 곱 연산(VMM) 수행 작업에 최적화 되어 있다.
마이크로칩의 memBrain 기술 평가 키트는 인텔리전트 하드웨어 코리아(Intelligent Hardware Korea, IHWK)가 엣지에서 추론 알고리즘을 실행하는 뉴로모픽 컴퓨팅 플랫폼의 최대 전력 효율성을 입증하기 위한 목적으로 설계되었다. 이를 통해 IHWK는 생성형 AI 모델, 자율 주행 자동차, 의료 진단, 음성 처리, 보안/감시 및 상업용 드론과 같은 애플리케이션을 위한 초저전력 아날로그 처리 장치(Analog processing unit) 개발을 최종 목표로 하고 있다.
인공지능(AI) 컴퓨팅과 관련 추론 알고리즘이 네트워크 엣지에 급속도로 도입됨에 따라 IHWK는 뉴로테크놀로지 디바이스 및 필드 프로그래머블 뉴로모픽 디바이스를 위한 뉴로모픽 컴퓨팅 플랫폼을 개발 중에 있다.
현재 엣지 추론을 위해 사용되는 뉴럴 모델은 프로세싱을 위해서만 5,000만 개 이상의 시냅스(가중치)를 필요로 하며, 순수 디지털 솔루션에만 요구되는 오프칩 DRAM의 대역폭 확보 또한 어려워 뉴럴 컴퓨팅에 병목 현상이 발생해 전체 컴퓨팅 성능이 저하될 수 있다.
반면에 memBrain 솔루션은 시냅스(가중치)를 온칩 플로팅 게이트에 초저전력 서브-임계 모드에서 저장하고, 동일한 메모리 셀을 사용하여 컴퓨팅을 수행하므로 전력 효율성 및 시스템 지연 시간을 크게 개선한다. 기존의 디지털 DSP 및 SRAM/DRAM 기반 접근 방식과 비교하면, 마이크로칩의 솔루션은 각 추론 결정 당 전력 사용량이 10~20배 더 낮고 전체 비용을 크게 절감한다.
IHWK는 APU 디바이스 개발 및 설계를 위해 대전에 있는 한국과학기술원(KAIST)과 서울 연세대학교와 협력하고 있다. 최종 APU는 추론 작업을 수행하는데 필요한 알고리즘을 최적화하여, 그 결과 배터리로 구동되는 디바이스에서 사용하기 위해 설계된 인메모리 컴퓨팅 솔루션 중 가장 우수한 와트당 20-80 테라OPS 범위에서 작동할 것으로 예상된다.
SST 라이선스 사업부 부사장 마크 라이튼(Mark Reiten)은 “마이크로칩의 memBrain 인메모리 컴퓨팅 기술은 네트워크 엣지에서 AI 처리를 수행할 때 발생하는 대용량 데이터 통신 병목 현상을 제거하기 위해 오프칩 메모리 솔루션 대신 검증된 NVM을 사용하며 이를 통해 일반적인 AI 프로세싱에서 발생하는 문제를 해결할 수 있었다”라며 “대학이자 얼리어답터 고객인 IHWK와의 협력을 통해 뉴럴 프로세싱에 대한 우리의 기술력을 입증하면서 대한민국 AI 산업 발전에 기여할 수 있게 돼 기쁘다”라고 말했다.
IHWK 윤상훈 지사장은 “한국은 AI 반도체 개발에 중요한 역할을 하고 있는 지역 중 하나다”라며 “비휘발성 및 신흥 메모리 분야의 전문가들은 인메모리 컴퓨팅 시스템을 개발하는데 있어 업계에서 검증받은 마이크로칩의 NVM 기술을 기반으로 한 memBrain 제품을 최적의 선택지로 꼽았다”고 말했다.
memBrain 솔루션의 프로세싱 요소 내에 뉴럴 모델을 영구적으로 저장하는 것은 실시간 뉴럴 네트워크 프로세싱을 위한 즉각적인(instant-on) 기능을 지원한다는 것을 뜻한다. IHWK는 슈퍼플래시 메모리의 플로팅 게이트 셀의 비휘발성을 활용, 고급 머신러닝 모델을 사용한 전력 엣지 컴퓨팅 장치에서 머신러닝 추론을 지원하는 새로운 벤치마크를 달성하고 있다.