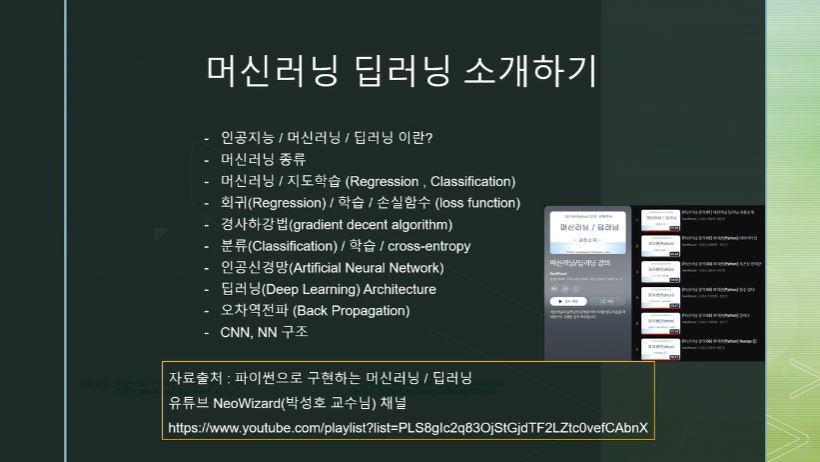
▲입문자를 위한 머신러닝&딥러닝 소개(캡처:e4ds EEWebinar)
“회귀 모델은 점수 예측, 분류는 합격 여부 예측”
2진 분류 모델, Logistic Regression 알고리즘 유용
머신러닝과 딥러닝이 생활 전반으로 확산되고 있는 가운데 인공지능 전문가들이 아닌 초급, 입문개발자들을 위한 원포인트 강의가 열렸다.
e4ds EEWebinar에서 ‘입문자를 위한 머신러닝&딥러닝 소개’ 웨비나 1편이 최근 공개됐다. e4ds 전자기술연구소에서 진행한 이번 웨비나는 e4ds 메이크에서 YOLO 버전8 객체 탐지를 주제로 콘테스트를 진행하는 가운데 인공지능 용어와 기본 개념을 소개하며 AI 비전공자 및 입문자용 교육을 진행했다.
입문자를 위한 머신러닝&딥러닝 소개 웨비나는 머신러닝과 딥러닝을 알아보고, △머신러닝과 지도학습 △회귀·학습·손실함수 △경사하강법 △인공신경망 △딥러닝 아키텍처 △오차역전파 △CNN·NN 구조 등에 대해 설명한다.
이날 웨비나 1편에서는 지도학습에서의 회귀 및 분류 모델에 대한 전반적인 설명과 손실함수, 경사하강법 등을 중심으로 강의가 진행됐다.
인공지능은 학습과 추론을 포괄하며 그 안에 머신러닝이 포함된다. 머신러닝은 데이터를 이용해데이터 특성과 패턴을 학습해 이를 바탕으로 미래값을 예측한다. 딥러닝은 머신러닝의 한 분야로서 신경망을 통해 학습하는 알고리즘의 집합이다.
머신러닝에는 △지도학습 △비지도학습 △강화학습이 있으며 전종천 책임연구원은 지도학습에 초점을 맞춰 설명했다. 지도학습엔 회귀(Regression) 모델과 분류(Classification) 모델로 나뉘며, 회귀 모델은 입력값에 대해 연속적인 출력값을 예측하는 것이며, 분류 모델은 입력데이터가 어떤 카테고리에 속하는지를 판별하는 것이다.
전 책임연구원은 “예컨대 회귀 모델은 공부시간에 따른 시험성적 점수를 예측하는 모델 개발에 적합하며, 분류 모델은 공부시간에 따른 시험의 합격 여부 등에 적합하다”고 덧붙였다.
회귀 모델은 오차가 가장 적게 나오는 가중치와 바이어스를 찾는 것이 중요한 요소로, 전 책임연구원은 “손실함수는 정답과 예측값과의 차이로 오차값을 구하는 함수인데, 가능하면 머신러닝에서 학습효과를 크게 주기 위해 제곱으로 계산해 오차를 더 큰 값으로 만든다”고 말했다.
이는 제곱에 의해 오차가 더 큰 값을 가지게 되며, 제곱을 하지 않을 경우 오차의 합이 0이 되는 문제도 방지할 수 있어 머신러닝에서 효과적인 학습이 가능한 것이다.
이어 전 책임연구원은 2진 분류 모델에서 사용되는 Logistic Regression 알고리즘을 설명했다. 예컨대 시험 합격 여부를 가르는 경계점을 찾는 데 Logistic Regression 알고리즘은 학습 데이터 분포에서 나타나는 최적의 직선을 찾고 이를 기준으로 분류해준다.
이러한 Logistic Regression 알고리즘은 분류 알고리즘 중에서도 정확도가 높아 딥러닝에서도 기본 컴포넌트로 활용되고 있는 것으로 알려져 있다.
한편, ‘입문자를 위한 머신러닝&딥러닝 소개’ 웨비나 2편이 오는 6일 e4ds EEWebinar에서 라이브로 진행될 예정이다.