[편집자주] 최근 온디바이스 AI 기반 제품들이 다양한 분야에서 빠르게 빌드업되고 있다. 이러한 상황에서 하드웨어 중심의 기업들이 AI 기능 구현을 쉽고 빠르게 달성해 제품 개발 기간을 단축시키고 선제적인 시장 진입을 지원하는 솔루션의 활용이 필요한 시점이다. 지난 15일 2024 스타트 온디바이스 AI(Start On-Device AI, 이하 2024 SODA) 컨퍼런스가 e4ds news 주관으로 개최되며 저전력·저사양 보드에 탑재하기 위한 AI 모델 경량화 및 최적화 솔루션에 대한 인사이트가 공유됐다.
2024 SODA에서 엣지 AI에 대해 발표한 ST마이크로일렉트로닉스의 문현수 과장과 함께 ST의 엣지 AI 기술과 개발 사례 등에 대해서 이야기를 나눴다.
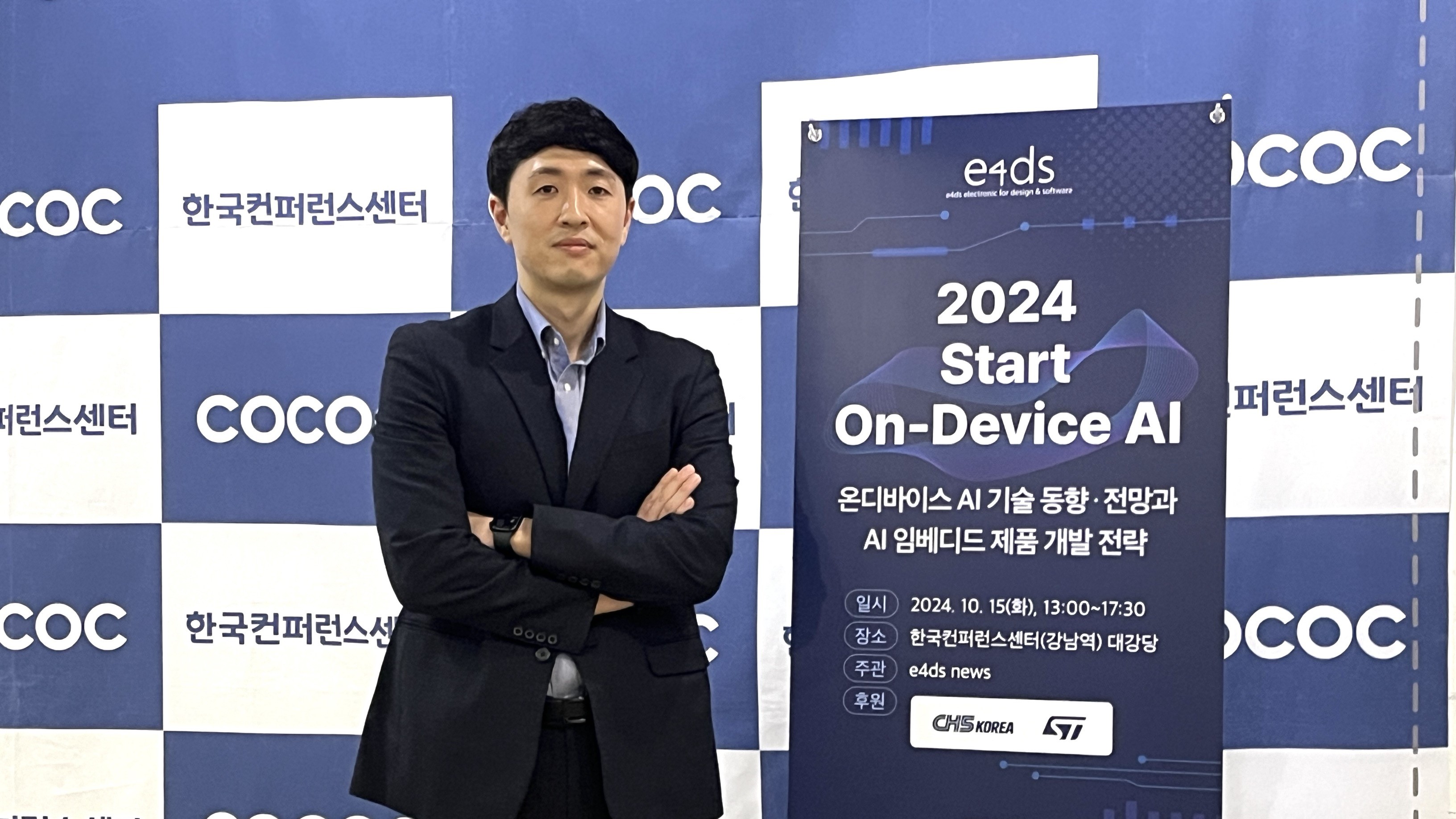
▲2024 SODA에 발표연사로 참여한 ST마이크로일렉트로닉스의 문현수 과장
엣지 AI 핵심, ML모델 손실 최소화한 경량화·엣지 HW
애프터 마켓 엣지 AI 기기, 투자 비용·전력소모 절감
2024 SODA 참여로 엣지 AI 무한 성장 가능성 재확인
[편집자주] 최근 온디바이스 AI 기반 제품들이 다양한 분야에서 빠르게 빌드업되고 있다. 이러한 상황에서 하드웨어 중심의 기업들이 AI 기능 구현을 쉽고 빠르게 달성해 제품 개발 기간을 단축시키고 선제적인 시장 진입을 지원하는 솔루션의 활용이 필요한 시점이다. 지난 15일 2024 스타트 온디바이스 AI(Start On-Device AI, 이하 2024 SODA) 컨퍼런스가 e4ds news 주관으로 개최되며 저전력·저사양 보드에 탑재하기 위한 AI 모델 경량화 및 최적화 솔루션에 대한 인사이트가 공유됐다.
2024 SODA에서 엣지 AI에 대해 발표한 ST마이크로일렉트로닉스의 문현수 과장과 함께 ST의 엣지 AI 기술과 개발 사례 등에 대해서 이야기를 나눴다.
■ ST의 엣지 AI기술과 솔루션에 대한 소개
ST는 MCU 및 MPU 제품과 같이 소규모의 메모리 사이즈와 연산 성능을 갖춘 소형 임베디드 플랫폼에서도 딥러닝을 포함한 머신 러닝 알고리즘을 프로세싱할 수 있도록 하드웨어와 소프트웨어를 제공한다.
STM32Cube.AI를 사용하면 호스트 PC에서 텐서플로우 라이트(TensorFlow Lite)와 같은 범용 프레임워크에서 만들어진 사전 학습된 딥러닝 모델을 C코드 기반의 모델로 변환해 STM32 제품에 적용할 수 있다.
나노엣지 AI 스튜디오(NanoEdge.AI Studio)는 머신 러닝에 대한 경험이나 지식이 없더라도 만들고 싶은 머신 러닝 모델에 필요한 입력 데이터셋만 준비할 수 있다면 데이터의 전처리, 머신 러닝 알고리즘의 추천과 학습 그리고 STM32 제품에 적용할 수 있는 코드까지 자동으로 생성해주는 AutoML 방식의 파이프라인을 제공한다.
STM32Cube.AI와 나노엣지 AI 스튜디오를 통해 STM32 제품을 사용하는 고객들이 빠르고 쉽게 머신 러닝 알고리즘을 STM32 제품에 적용할 수 있다.
■ 시장/업계의 주요 요건과 경쟁사 제품(또는 기존 제품) 대비 차별성 및 특장점은?
엣지 AI를 구성하는 하드웨어의 경우, 제조사도 다양하고 제품의 종류와 제공하는 솔루션이 매우 다양하다. 엣지 AI의 핵심은 사전 학습된 머신 러닝 모델의 손실을 최소화하는 경량화와 엣지에서 머신 러닝 모델의 연산을 가속화시킬 수 있는 하드웨어를 구성하는 것이다.
ST의 STM32 제품군은 저전력을 기반으로 다양한 라인업을 구성하고 있어 낮은 소비 전력으로 엣지 컴퓨팅을 수행할 수 있다. 특히, STM32Cube.AI와 나노엣지 AI 스튜디오의 소프트웨어 툴을 사용하면 사용자는 쉽고 빠르게 머신 러닝 모델을 STM32 제품에 적용할 수 있으며, 머신 러닝 모델의 손실을 최소화하는 경량화에 필요한 다양한 옵션들도 제공되고 있다.
■ 주요 적용 애플리케이션 및 산업 현장과 일상 생활에서 접할 수 있는 대표적인 사용 사례 소개
엣지 AI는 작게는 △웨어러블 △가전기기 △로봇에 이용되고 있으며, 크게는 △산업용 기기 △스마트 홈 △스마트 빌딩 △스마트 시티 등 다양한 분야에 적용되고 있다.
대표적으로 모터에 모듈을 추가 설치해 구현되는 예측유지보수가 있다. 그 작동 원리는 이런 모듈에 MEMS 센서로 모터의 진동을 감지하는데, 사람이 감지할 수 없는 미세한 비정상 진동도 감지한다.
이런 미세한 모터의 진동 데이터로 고장이 나기 전에 유지보수시기를 알려주는 시스템이 있다. 이처럼 기존 기기에 간단히 엣지 AI 모듈을 추가한 애프터 마켓 엣지 AI 기기는 투자 비용이 적고 전력소모가 매우 적다.
일반적인 가전제품으로는 드럼 세탁기가 있다. 엣지 AI를 적용하면 에너지 효율을 최대 40%까지 높일 수 있다. 드럼세탁기는 회전하는 드럼으로 인해 세탁물의 실제 무게 측정이 어렵지만 모터의 처음 동작 시 전류를 측정해 세탁물의 무게를 예측하고 그 무게에 맞는 세제, 물, 세탁 시간을 결정해 에너지 효율을 최적화하는 AI 시스템이다. 효율이 더 높고 에너지 소비를 줄이며, 유지보수 비용도 절감할 수 있다.
보안 분야에서는 CCTV를 이용해 STM32N6 MCU로 클라우드를 사용하지 않고 기기 자체가 독립적으로 비디오 이미지 인식이 가능하다. △자동차 △트럭 △버스 △오토바이 △보행자를 자동으로 인식하고 색깔별로 보여준다.
5M픽셀 HD 화질 18 FPS로 동작되고 전력 사용량은 0.5와트, 전력사용 비용은 하루 1센트 이하에 불과하다. AI 기능이 추가된 CCTV 시스템을 스마트 팜에 도입하면 농장에서 야생동물 출현을 자동으로 인식하고 농장주에게 알림을 준다.
.jpg)
▲2024 SODA에서 세션 발표 중인
문현수 과장의 모습
■ 솔루션의 향후 발전 방향과 목표 시장에 대해 설명
엣지 AI는 다양한 산업 분야에서 혁신적인 변화를 이끌고 있으며, 실시간 데이터 처리, 향상된 유지보수, 안전성 강화 등 여러 측면에서 사용자 경험을 크게 향상시킬 수 있다. ST는 AI 기능이 탑재되는 온디바이스 AI 시장을 겨냥해 MCU 기능 업데이트를 지속적으로 진행하고 있다.
또한, ST는 보안 성능을 강화한 신제품으로 온디바이스 AI 시장을 집중적으로 공략할 예정이다. 내년부터 미국과 유럽에서 보안 규격이 강화되는데, 이번에 출시한 저전력 고성능 MCU 제품군은 전력 효율과 성능뿐만 아니라 보안 기능까지 향상됐다. 헬스케어와 도어락, 스마트 홈뿐만 아니라 각종 고성능 AI 분야에서도 적용을 확대해 나갈 예정이다.
■ ST의 온디바이스 AI 관련 에코시스템/협력 현황
엣지 AI는 많은 장점이 있지만, AI 개발자들이 시작부터 끝까지 다양한 개발을 진행해야 한다. 이러한 엣지 AI의 개발을 위해서는 다양한 하드웨어와 소프트웨어 툴이 필요하며, 에코시스템을 통해 이를 해결할 수 있다.
ST는 매우 광범위하고 포괄적인 에코시스템을 운영하며, 이를 통해 보드, 소프트웨어, 드라이버, 라이브러리 등을 지원한다. 애플리케이션 개발은 고객사가 가장 잘 알고 있기 때문에 ST는 고객이 애플리케이션을 잘 개발할 수 있도록 툴을 제공하는 역할을 한다.
이러한 에코시스템을 통해 개발자들은 다양한 환경에서 효율적으로 엣지 솔루션을 간편하게 개발하고 개발시간을 단축할 수 있다.
■ 2024 SODA에서 발표한 내용과 관련해 행사 참여 소감은?
2024 SODA 행사 참여를 통해 엣지 AI의 무한한 성장 가능성을 다시 한번 확인할 수 있었다. 엣지 AI 플랫폼의 하드웨어를 제조하는 제조사 입장에서 빠르게 발전하는 엣지 AI기술이 STM32 제품과 결합되었을 때의 강점을 고객들께 직접 소개할 수 있는 소중한 자리였기 때문에 더욱 의미가 있었다.
ST는 고객의 제품이 머신러닝 모델을 통해 더 빠르고 스마트하게 세상과 연결될 수 있도록 언제나 최선의 지원을 아끼지 않을 예정이다.