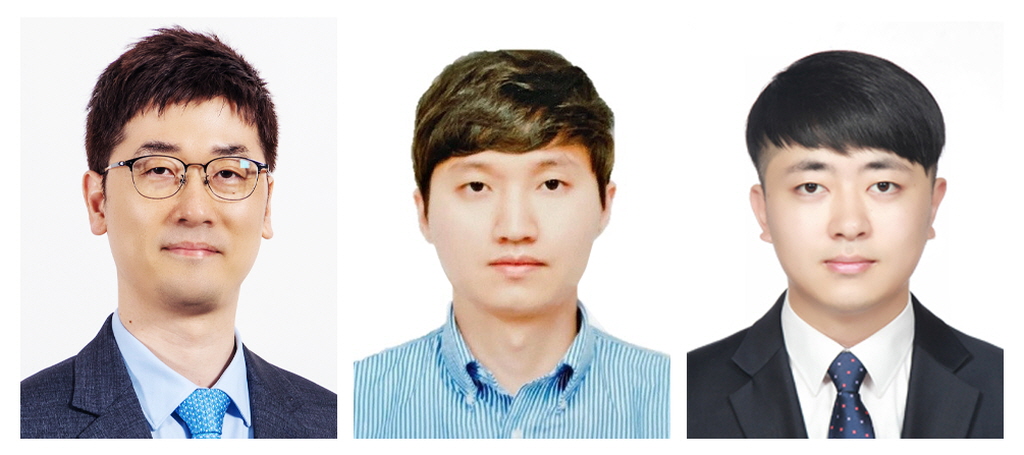
▲(왼쪽부터)김성일 UNIST 교수, 임동영 교수, 제 1저자 오용경 연구원
UNIST, 데이터 드리프트에 강건한 시계열 학습 기술 개발
인공지능 모델의 성능을 저하시키는 현상에 효과적으로 대응할 수 있는 학습 기술이 개발됐다. 국내 산업에서의 인공지능 활용 가능성 제고와 성능 강화에 기여할 수 있을 것으로 기대된다.
UNIST (총장 이용훈) 산업공학과 및 인공지능대학원 김성일, 임동영 교수팀은 ‘데이터 드리프트에 강건한 시계열 학습 기술’을 개발했다.
시계열 데이터는 시간 순서에 따라 일정 주기를 가지고 연속적으로 수집된 데이터를 말한다. 금융, 경제, 교통, 농업, 제조, 헬스케어 등 각종 산업에서 사용되는 수많은 데이터가 시계열 형태를 가지고 있다.
시계열 데이터는 데이터 발생에 영향을 주는 외부 요인들이 변함에 따라 ‘데이터 드리프트’라는 현상이 발생한다. 데이터 드리프트는 인공지능 모델이 훈련에 사용한 데이터 실제 운영 환경의 데이터가 달라지는 것을 말한다.
김성일 교수는 “데이터 드리프트가 발생할 경우 시계열 학습 인공지능 모델의 성능이 저하된다”며 “각종 산업 등에서 시계열 데이터 활용을 어렵게 만드는 고질적 문제다”고 덧붙였다.
연구팀은 이런 문제를 효과적으로 대응할 수 있게 하는 Neural SDEs(Stochastic Differential Equations) 기반의 강건한 신경망 구조 설계에 대한 방법론을 개발했다.
Neural SDEs는 잔차 신경망 모델의 연속된 버전인 Neural ODEs를 확장한 모델이다. 연구팀은 데이터 드리프트 현상에서도 강건함을 유지할 수 있는 시계열 Neural SDEs 모델 설계 방법론에 대한 이론적 근거를 제시했다.
연구팀은 방법론에 따라 설계한 세 가지 Neural SDEs 모델 Langevin-type SDE, Linear Noise SDE, Geometric SDE을 선보였다. 제안된 모델들은 데이터 드리프트 현상이 일어난 데이터셋에서 보간, 예측, 분류 등의 다양한 작업을 수행할 때 안정적이며 우수한 성능을 보였다.
데이터 드리프트 현상이 발생했을 때, 이를 빠르게 포착하고 데이터를 재구성해 재학습하는 일련의 엔지니어링 과정은 큰 시간과 비용이 수반됐다. 연구팀은 인공지능을 처음부터 데이터 드리프트 현상에 강건할 수 있도록 만드는 데에 필요한 기술을 이론적, 실험적으로 모두 검증한 것이다.
임동영 교수는 “최근 동적 데이터 환경에 따른 데이터 드리프트로 인해 시계열 인공지능 모델의 성능이 저하되는 사례들이 빈번하다”며 “이 연구는 처음부터 드리프트에 강건하도록 인공지능을 훈련시킬 수 있도록 하는 방법론을 개발, 이의 성능을 이론적, 실험적으로 검증했다는 데에 의의가 있다”고 전했다.
제 1저자 오용경 연구원은 “이번 연구를 통해 시계열 데이터 드리프트로 인한 인공지능의 성능이 저하되는 것을 막기 위한 신경망 구조 설계 방법론을 개발했다”며 “앞으로 개발된 기술과 연계된 시계열 데이터 드리프트 감시 기술, 학습 데이터 재구성 기술 등을 지속적으로 개발해 국내 다양한 기업들이 활용할 수 있도록 할 계획이다”고 밝혔다.
이번 연구는 세계적인 권위의 국제 학회인 ICLR(International Conference on Learning Representations)에 상위 5%에 해당하는 spotlight 논문으로 선정돼 오는 5월 오스트리아 빈에서 발표될 예정이다. 또한, 한국보건산업진흥 바이오메디컬 글로벌 인재양성사업, 정보통신기획평가원 인공지능대학원사업, 과학기술정보통신부 한국연구재단 기본 연구 및 인간중심-탄소 중립 글로벌 공급망 연구센터의 지원으로 이뤄졌다.