UNIST(총장 박종래) 지구환경도시건설공학과 임정호 교수팀이 1년 뒤의 북극 해빙 농도를 6% 이내 오차 정확도로 예측할 수 있는 인공지능 모델을 개발하며, 북극 해빙 변화에 대한 중장기 예측 정보 제공이 가능해져 북극 항로 개발, 해양 자원 탐사 등에 도움이 될 전망이다.
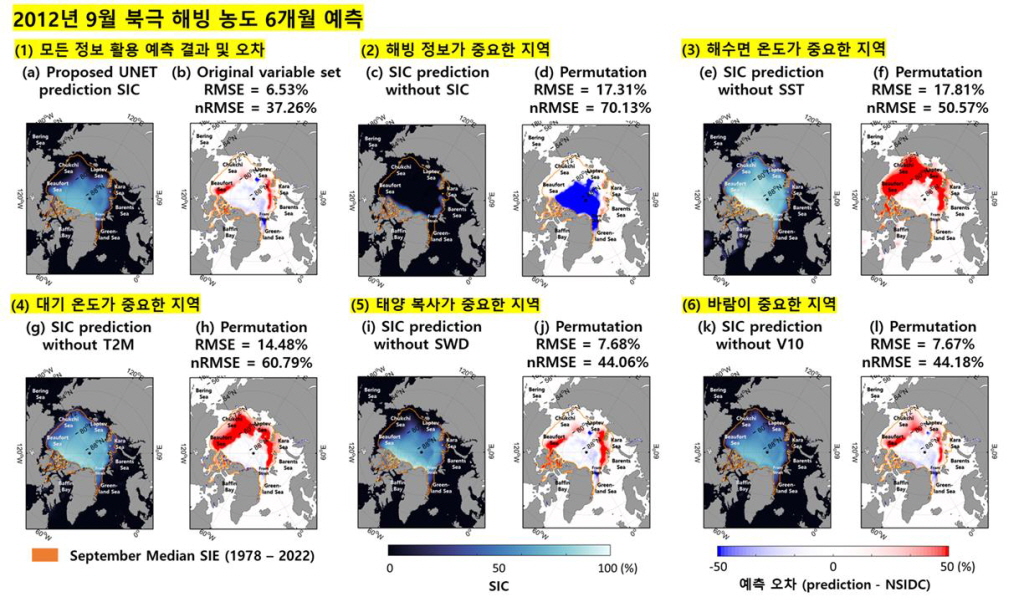
▲예측 결과의 성능 차이를 활용해 각 기후요인이 해빙농도 예측에 미치는 영향 분석
UNIST 임정호 교수팀, UNET 딥러닝 기반 해빙 농도 예측 모델 개발
최대 1년 뒤의 북극 해빙 변화를 예측할 수 있는 인공지능(AI) 모델이 나오며, 중장기 예측 정보 제공이 가능해져 북극 항로 개발, 해양 자원 탐사 등에 도움이 될 전망이다.
UNIST(총장 박종래)는 최근 지구환경도시건설공학과 임정호 교수팀이 1년 뒤의 북극 해빙 농도를 6% 이내 오차 정확도로 예측할 수 있는 인공지능 모델을 개발했다고 밝혔다.
해빙 농도는 단위면적에서 얼음이 덮인 영역의 비율을 말한다.
연구팀은 유넷(UNET)을 활용해 과거 북극 해빙 농도의 변화 패턴과 기온 및 수온, 태양 복사량, 바람 같은 주요 기후 요인 간의 복잡한 관계를 학습시켜 이 같은 AI 모델을 개발했다.
UNET은 AI가 위성영상과 같은 이미지 데이터 간의 관계를 학습하는 딥러닝 알고리즘 중 하나다.
개발된 모델은 중장기 예보 정확도가 높았다. AI 모델의 예측값과 과거 실제 해빙 농도 값을 비교하는 방식으로 정확도를 평가해 본 결과, 3개월, 6개월, 12개월 예측에서 모두 6% 미만의 평균 예측 오차를 기록했다.
기존 모델은 예측 기간이 길어질수록 평균 예측 오차가 증가했다.
또한 이 모델은 이례적으로 해빙이 급격히 감소했던 상황에서도 안정적인 예측 성능을 보였다.
2007년, 2012년 여름과 같이 해빙이 급격히 녹아버린 경우, 기존 모델은 17.35%의 평균 예측 오차를 기록한 반면에 개발된 AI 모델은 7.07%의 평균 예측 오차를 기록해 평균 예측 오차 값이 절반 이하로 줄었다.
연구팀은 해빙 농도 중·장기 예측에서 중요한 역할을 하는 기후 요소들도 밝혀냈다.
UNET 모델 예측 결과 간 차이를 분석한 결과, 얼음 두께가 얇은 해빙 가장자리에서는 태양복사열과 바람이 주요 변수로 작용했다.
임정호 교수는 “기존 물리 기반 모델의 한계를 극복하고, 다양한 환경 요인들이 북극 해빙 변화에 미치는 복합적인 영향을 규명한 연구”라며 “북극 항로 개발, 해양 자원 탐사, 기후 변화 대응 정책을 수립하는 데 도움이 될 것”이라고 밝혔다.
이번 연구는 국제학술지인 환경원격탐사(Remote Sensing of Environment)에 12월11일 자로 온라인 게재됐으며, 극지연구소, 해양수산부의 지원을 받아 이뤄졌다.